M.Tech. Programmes
DEGREE PROGRAMMES M.Tech. Data Science and Engineering
M.Tech. Data Science and Engineering
According to LinkedIn's Emerging Jobs report, Data Science has emerged as the fastest-growing job globally, with a remarkable growth rate of over 650% since 2012. Moreover, the Data Science market is predicted to follow an upward trajectory, increasing from USD 37.9 billion in 2019 to a projected value of USD 230.80 billion by 2026.
Prepare for a career in Data Science with India’s most comprehensive and world-class M.Tech. Data Science & Engineering Programme without taking a career break. This four-semester programme by BITS Pilani WILP enables Software and IT professionals to build stellar skill set required to advance their career as a Data Analyst, Data Engineer, Data Architect, and Data Scientist, etc.
Download Brochure Apply Now- Programme Highlights
- UGC Approval
- Programme Curriculum
- Learning Methodology
- Eligibility Criteria
- Fee Structure
- How to Apply
Programme Highlights
-
M.Tech. Data Science and Engineering is a BITS Pilani Work Integrated Learning Programme (WILP). BITS Pilani Work Integrated Learning Programmes are UGC approved.
-
The programme is of four semesters, with online classes conducted mostly on weekends or after business hours. You can pursue the programme without any career break.
-
Offers the most comprehensive Data Science Curriculum for working professionals.
-
The programme has an unmatched range & depth, and covers fundamentals to advanced skill & knowledge areas associated with the domain of Data Science.
-
Aimed at transitioning software & IT professionals into Data Science careers tracks closest to their interest/passion.
-
Curriculum maps knowledge and skill areas required to perform popular Data Science job roles such as Data Analyst, Data Engineer, Data Architect, and Data Scientist, etc.
-
The programme offers a set of core courses and elective courses, allowing students to specialize in Data Management for Machine Learning, Ethics for Data Science, Optimization Techniques for Analytics, Natural Language Processing, etc.
-
The programme makes use of Tools and Technologies. These include Apache Spark, Apache Storm for Big Data Systems/ Real time Processing; Tableau for data visualisation; Tensorflow for Deep Learning; Various Packages within Python for data processing, machine learning, data visualization etc.
-
The Dissertation (Project Work) in the final semester enables students to apply concepts and techniques learned during the programme.
-
The programme uses a Continuous Evaluation System that assesses the learners over convenient and regular intervals. Such a system provides timely and frequent feedback and helps busy working professionals stay on course with the programme.
-
The education delivery methodology is a blend of classroom and experiential learning. Experiential learning consists of lab exercises, assignments, case studies and work-integrated activities.
-
Participants who successfully complete the programme will become members of an elite & global community of BITS Pilani Alumni
-
Option to submit fee using easy-EMI with 0% interest and 0 down payment .
UGC Approval
BITS Pilani is an Institution of Eminence under UGC (Institution of Eminence Deemed to be Universities) Regulations, 2017. The Work Integrated Learning Programmes (WILP) of BITS Pilani constitutes a unique set of educational offerings for working professionals. WILP are an extension of programmes offered at the BITS Pilani Campuses and are comparable to our regular programmes both in terms of unit/credit requirements as well as academic rigour. In addition, it capitalises and further builds on practical experience of students through high degree of integration, which results not only in upgradation of knowledge, but also in up skilling, and productivity increase. The programme may lead to award of degree, diploma, and certificate in science, technology/engineering, management, and humanities and social sciences. On the recommendation of the Empowered Expert Committee, UGC in its 548th Meeting held on 09.09.2020 has approved the continued offering of BITS Pilani’s Work Integrated Learning programmes.
Programme Curriculum
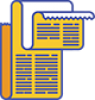
Participants need to take at least 12 courses towards coursework, and complete one Project/ Dissertation. The coursework requirement for the programme would consist of a set of core courses and electives. Core courses are compulsory for all participants, while electives can be chosen based on individual learning preferences.
First Semester
-
Mathematical foundations for Data Science
-
Introduction to Data Science
-
Computer Organization and Software Systems
-
Data Structures and Algorithm Design
Second Semester
-
Introduction to Statistical Methods
-
Elective 1
-
Elective 2
-
Elective 3
Third Semester
-
Big Data Systems
-
Elective 4
-
Elective 5
-
Elective 6
Fourth Semester
-
Dissertation
Electives
-
Data Warehousing
-
Graphs – Algorithms and Mining
-
Deep Learning
-
Probabilistic Graphical Models
-
Ethics for Data Science
-
Optimization Techniques for Analytics
-
Data Management for Machine Learning
-
Natural Language Processing
-
Design of Experiments for Data Science
-
Information Retrieval
-
Data Visualization and Interpretation
-
Stream Processing and Analytics
-
Artificial and Computational Intelligence
-
Machine Learning #*
-
Applied Machine Learning
Note: #*Machine Learning course is a prerequisite for Deep Learning elective course
Electives finally offered will be at the discretion of the BITS Pilani, and will be decided in consultation with HCL. Offered electives will be made available to enrolled students at the beginning of each semester.
Learning Methodology

ATTEND ONLINE LECTURES OVER WEEKENDS
-
Lectures are conducted live via online classes. These lectures can be attended via the internet using a computer from any location. These online classrooms offer similar levels of interactivity as regular classrooms at the BITS Pilani campus.
-
The class schedule is announced within 1 week of completion of the admission process.
-
The online lectures are conducted usually over weekends for a total of 7-8 hours per week. If you miss a lecture, you can also access the recorded lecture on the internet.
-
Lectures are conducted on Sat/Sun as per Indian Standard Time.

PROJECT WORK
During the final semester participants carryout a semester-long intensive project work applying the various concepts learnt throughout the program guided by the organisation mentor and supervisor. Participants are provided access to virtual labs where applicable, and faculty expertise to support the project work.

EXPERIENTIAL LEARNING & LABS
The programme emphasises on Experiential Learning that allows learners to apply concepts learnt in classroom in simulated and real work situations. This is achieved through:
-
Tools & Technologies: Apache Spark, Apache Storm for Big Data Systems/ Real time Processing; Tableau for data visualisation; Tensorflow for Deep Learning and various Python libraries for data processing, machine learning, OpenCV for computer vision, NLTK for NLP etc..
CASE STUDIES AND ASSIGNMENTS: Carefully chosen real-world cases & assignments are both discussed and used as problem-solving exercises during the programme.
DISSERTATION/ PROJECT WORK: The fourth semester offers an opportunity for learners to apply their knowledge gained during the programme to a real-world like complex project. The learner is expected to demonstrate understanding of vital principles learnt across semesters and their ability to successfully apply these concepts.
SUPPLEMENTARY LEARNING: In addition to live weekly online lectures, supplementary live online sessions will be organised periodically comprising of tutorials, doubt-clearing interactions, and industry talks (18-20 hours per semester).

CONTINUOUS ASSESSMENT
The learners' performance is assessed continuously throughout the semester using various tools such as quiz, assignments, mid-semester and comprehensive exams. The assessment results are shared with the learners to improve their performance. Each course will entail a minimum of 1 Assignment/ Quiz, a Mid-semester exam and a final Comprehensive exam. Your semester calendar will indicate the dates of the Mid-semester and Comprehensive exam.Online Exams facility available.
Typically, a Mid-semester or Comprehensive examination for a course is for 2-3 hours duration. The examinations are typically conducted over a weekend, i.e. Saturday and Sunday.
Eligibility Criteria

Employed professionals holding B.Tech. / B.E. / M.Sc. / MCA or equivalent in relevant disciplines with at least 60% aggregate marks + Any full-time employee who has received the first appraisal rating of “Good Performance” or above in the last annual performance appraisal cycle are eligible to apply. Applicants should possess basic programming knowledge and adequate background in Mathematics.
The above are only the minimum criteria to apply. The final decision to offer admission to an applicant rests with BITS Pilani which will be made based on an overall review of your application information.
It is strongly advised to refer and check HCL's policy details and other Eligibility Criteria of the programme before applying, as all fees are non-refundable.
The information contained in this electronic communication is intended solely for the individual(s) or entity to which it is addressed. It may contain proprietary, confidential and/or legally privileged information. Any review, retransmission, dissemination, printing, copying or other use of, or taking any action in reliance on the contents of this information by person(s) or entities other than the intended recipient is strictly prohibited and may be unlawful. If you have received this communication in error, please notify us by responding to this email or telephone and immediately and permanently delete all copies of this message and any attachments from your system(s). The contents of this message do not necessarily represent the views or policies of BITS Pilani
Fee Structure

-
The following fees schedule is applicable for candidates seeking new admission during the academic year 2024-25
Application Fees (one time) : INR 1,500
Admission Fees (one time) : INR 16,500
Semester Fees (per semester) : INR 71,750
-
The one-time Application Fee is to be paid at the time of submitting the Application Form through the Online Application Centre.
-
Admission Fee (one-time) and Semester Fee (for the First Semester) are to be paid together once admission is offered to the candidate. Thus, a candidate who has been offered admission will have to pay Rs. 88,250/-. You may choose to make the payment using Netbanking/ Debit Card/ Credit Card through the Online Application Centre.
-
Semester Fee for subsequent semesters will only be payable later, i.e. at the beginning of those respective semesters.
-
Any candidate who desires to discontinue from the programme after confirmation of admission & registration for the courses specified in the admit offer letter will forfeit the total amount of fees paid.
-
All the above fees are non-refundable.
How to Apply
-
Click here to visit the Online Application Center. Create your login at the Online Application Center by entering your official HCL Email ID only and create a password of your choice. Once your login has been created, you can anytime access the Online Application Center using your official email ID and password.
-
Begin by clicking on Step 1 - ‘Fill/ Edit and Submit Application Form’. This will enable you to select the programme of your choice. After you have chosen your programme, you will be asked to fill your details in an online form. You must fill all details and press ‘Submit’ button given at the bottom of the form.
-
Now, click on 'Pay Application Fee’ to pay INR 1,500/- using Netbanking/ Debit Card/ Credit Card
-
Finally, click on 'Upload & Submit All Required Documents’. This will allow you to upload one-by-one all the mandatory supporting documents such academic certificates and transcripts, photograph, etc. and complete the application process. Acceptable file formats for uploading these documents are .DOC, .DOCX, .PDF, .ZIP and .JPEG
-
Upon receipt of your Application Form and all other enclosures, the Admissions Cell will scrutinise them for completeness, accuracy and eligibility.
-
Admission Cell will intimate selected candidates by email within two weeks of submission of application with all supporting documents. The selection status can also be checked by logging in to the Online Application Centre.